Systemic Risk for Privacy in Online Interaction
This project is related to our research line: Online Social Networks
Duration 12 months (January 2017 - December 2017)
Funding source ETH Zurich Foundation, through ETH Risk Center
The goal of this project is to understand the systemic dimension of privacy risks emerging from online interaction. Today, digital traces generated by millions of users allow to infer private attributes of individuals that may not even use online media. This poses a considerable risk to privacy which needs to be conceptualized, quantified and measured.
Hence, as a first step, we will explore ways to construct shadow profiles, i.e. aggregated information about individuals that was not provided by them, but inferred from the information disclosed by other users and their interactions. This allows us to reveal the conditions under which privacy risks can occur, to eventually mitigate them.
As a second step, we will developmodeling framework to test how social mechanisms are able to exacerbate this privacy risk. For example, the decisions of others to make their information public can be also driven by herding effects. Hence, in social networks there is an impact of the collective dynamics on the degree of privacy risk.
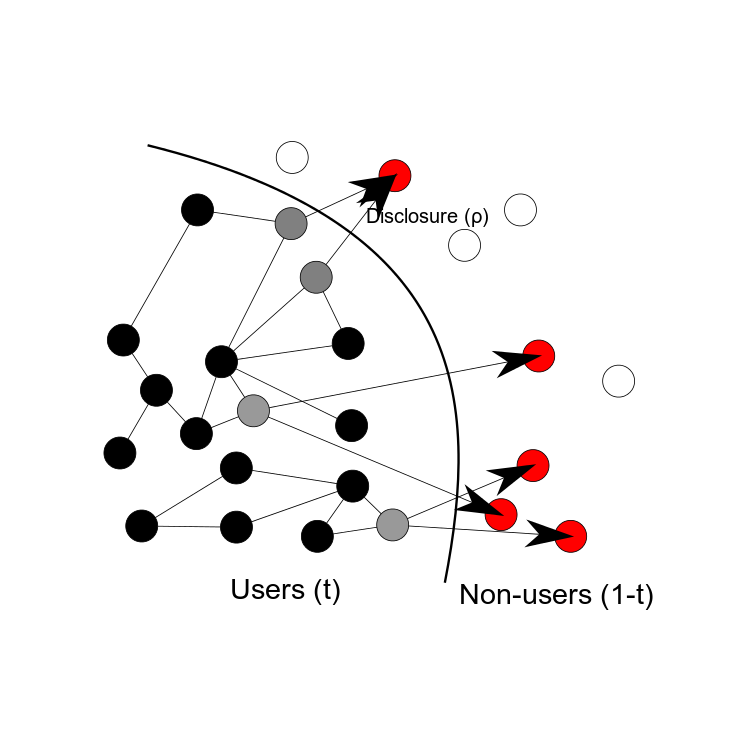
Figure: Schema of a full shadow profile construction problem (adapted from Sarigöl, Emre and Garcia, David and Schweitzer, Frank, 2014, "Online Privacy as a Collective Phenomenon")
Eventually, we want to explore how the increase of privacy risk leads to an increasing risk of the social network to collapse, because users may decide to leave or to not join the network. This implies to understand the systemic feedback between individual decisions and the resilience of the network, and has potential impact to help users to manage privacy, to inform policy makers in regulating online data use, and to aid online community designers to achieve a balance between information access and privacy.
With our research we aim at contributing to the following research questions:
- How is the decision of a user to not share private information affected by the online behavior and the connections of others?
- What is the tipping point beyond which individuals have a negligible privacy even if they did not reveal personal information, because their shadow profile
can be completed by social inference?
- If the loss of privacy can be perceived by users, how will that affect the resilience of an online social network?
Selected publications
Understanding Popularity, Reputation, and Social Influence in the Twitter Society
|
[2017]
|
Garcia, David;
Mavrodiev, Pavlin;
Casati, Daniele;
Schweitzer, Frank
|
Policy & Internet
|
more» «less
|
Abstract
The pervasive presence of online media in our society has transferred a significant part of political deliberation to online forums and social networking sites. This article examines popularity, reputation, and social influence on Twitter using large-scale digital traces from 2009 to 2016. We process network information on more than 40 million users, calculating new global measures of reputation that build on the D-core decomposition and the bow-tie structure of the Twitter follower network. We integrate our measurements of popularity, reputation, and social influence to evaluate what keeps users active, what makes them more popular, and what determines their influence. We find that there is a range of values in which the risk of a user becoming inactive grows with popularity and reputation. Popularity in Twitter resembles a proportional growth process that is faster in its strongly connected component, and that can be accelerated by reputation when users are already popular. We find that social influence on Twitter is mainly related to popularity rather than reputation, but that this growth of influence with popularity is sublinear. The explanatory and predictive power of our method shows that global network metrics are better predictors of inactivity and social influence, calling for analyses that go beyond local metrics like the number of followers.
Online Privacy as a Collective Phenomenon
|
[2014]
|
Sarigol, Emre;
Garcia, David;
Schweitzer, Frank
|
In Proceedings of the 2nd Conference on Online Social Networks 2014
|
more» «less
|
Abstract
The problem of online privacy is often reduced to individual decisions to hide or reveal personal information in online social networks (OSNs). However, with the increasing use of OSNs, it becomes more important to understand the role of the social network in disclosing personal information that a user has not revealed voluntarily: How much of our private information do our friends disclose about us, and how much of our privacy is lost simply because of online social interaction? Without strong technical effort, an OSN may be able to exploit the assortativity of human private features, this way constructing shadow profiles with information that users chose not to share. Furthermore, because many users share their phone and email contact lists, this allows an OSN to create full shadow profiles for people who do not even have an account for this OSN. We empirically test the feasibility of constructing shadow profiles of sexual orientation for users and non-users, using data from more than 3 Million accounts of a single OSN. We quantify a lower bound for the predictive power derived from the social network of a user, to demonstrate how the predictability of sexual orientation increases with the size of this network and the tendency to share personal information. This allows us to define a privacy leak factor that links individual privacy loss with the decision of other individuals to disclose information. Our statistical analysis reveals that some individuals are at a higher risk of privacy loss, as prediction accuracy increases for users with a larger and more homogeneous first-and second-order neighborhood of their social network. While we do not provide evidence that shadow profiles exist at all, our results show that disclosing of private information is not restricted to an individual choice, but becomes a collective decision that has implications for policy and privacy regulation.
|